Small flying insects have a lot to tell us about acquiring and processing data. As they fly, they make adjustments to counteract wind gusts, and they do it faster than any supercomputer. How they do this is still not fully understood, but UW biologist Bing Brunton and others are coming closer to finding answers.
What first intrigued Brunton, assistant professor of biology, is how flying insects can do so much with seemingly so little. They are able to make complex adjustments in flight based on inputs from a small number of well-placed sensors on their wings that collect very specific data.
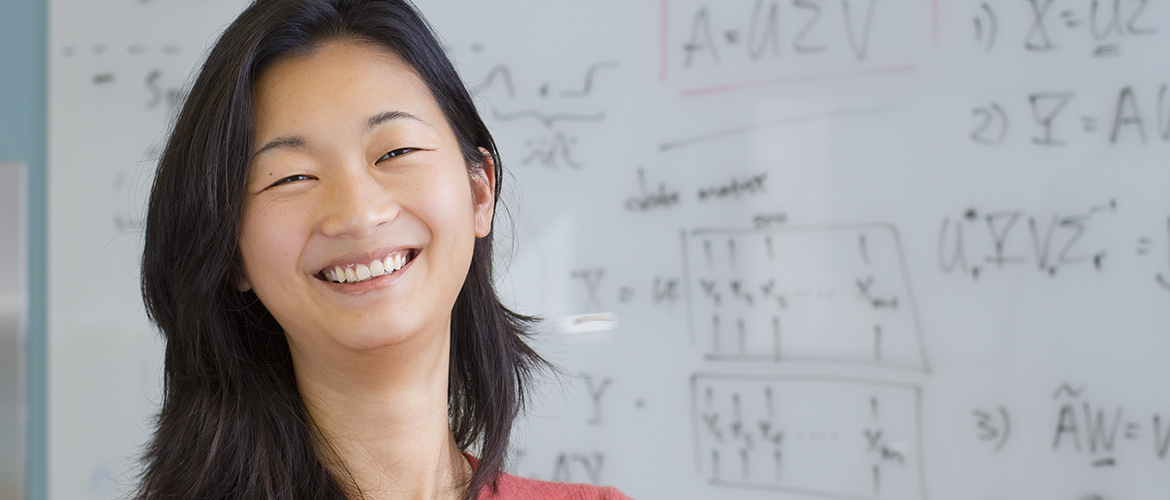
“Big data is all the rage,” says Brunton. “There is tendency to say that more data is always better. That’s true to a certain extent, but it’s not always true. Small flying insects demonstrate that sometimes less is better, if you are collecting the right data.”
Because flying insects make instantaneous adjustments while flying, their sensors must provide only the most pertinent information. Extraneous data would slow them down. They also face constraints that limit the number of sensors they can support. There’s limited real estate on their wings, and there’s an energy cost to growing sensors and analyzing the information they provide. Like those flying insects, technology also has constraints, such as limited resources for gathering and analyzing data.
Small flying insects demonstrate that sometimes less is better, if you are collecting the right data.
To address these constraints, Brunton first turned to mathematics, working with faculty in the Department of Applied Mathematics to develop a “sparse sensor” algorithm that favors smart data over big data. Then she tapped into ongoing neuroscience research in the Department of Biology — studies of the aerial prowess of hawk moths — to study how sparse sensors work in the natural world. Key collaborators in this work include Tom Daniel in Biology, Steve Brunton in Mechanical Engineering, and Nathan Kutz in Applied Mathematics.
Brunton and her team developed computer models of a hawk moth in flight, attempting to understand how moths adjust for external factors like gusts of wind. The team homed in on specific sensors on the moth’s wings — mechanoreceptor neurons — that produce signals, or data, that the moth requires. The team then devised a neural-inspired filter to isolate and study those signals. They discovered that the neurons measure a very subtle bending or rotation of the moth’s wing caused by the wind, and also track how that bending or rotation changes over time.
That the neurons provide time measurements is worth noting, says Brunton, since many statistical and computer science methods ignore time as a consideration. She believes that approach needs to change. “In my lab, we’re really interested in building techniques that are aware of correlations and relationships both across space and across time simultaneously,” Brunton says. “It’s something that living systems naturally do. The moth is just one example of that.”
While Brunton’s findings provide clues to moths’ aerial talents, there are many questions she still hopes to answer. Her team has a sense of how a moth might detect wind gusts, but not how the neurons then tell the moth to respond to that input. And they still don’t fully understand how moths accomplish so much with minimal data, or sparsity. But they’re getting closer.
“What’s really exciting is that there’s now a collision of different fields coming together around the ideas of sparsity and parsimony,” says Brunton. “I think it’s a ‘right place, right time’ kind of thing. We are using computational tools to understand neuroscience, and we can learn from neuroscience — from the way that animals do all the remarkable things they do — to help us build better technologies. I’m really excited about where these ideas are headed next.”
More Stories
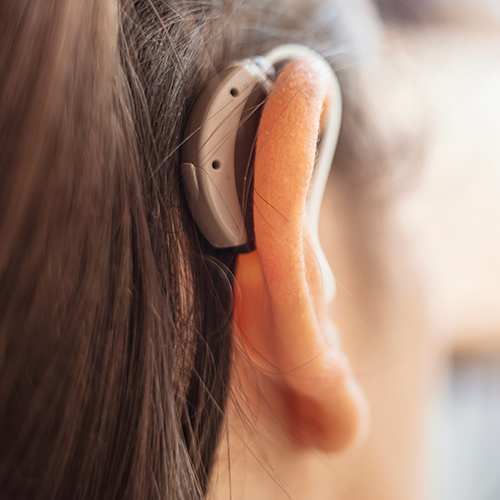
An Earful of AI
Hearing aid technology is improving all the time with the help of AI, thanks to researchers like Shen Yi, professor of speech & hearing sciences.
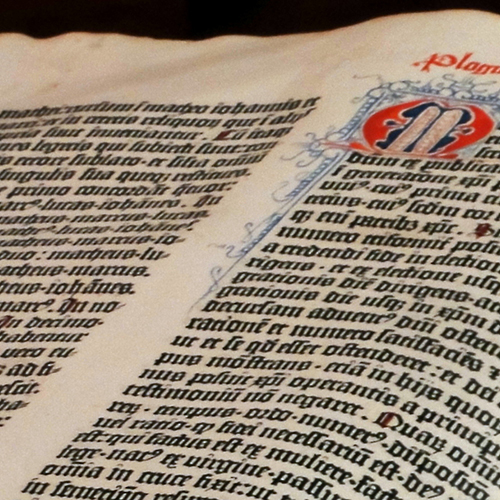
The Evolution of the Book
As books evolve with new technologies, Geoffrey Turnovsky, professor of French, explores the history of texts — and the reading experience.

You Discover You're on Camera. Now What?
A growing number of people use surveillance cameras inside their home. Interaction design professor James Pierce explores how others spending time in the home are affected.