If Siri has ever misunderstood your straightforward question, or your smartphone has autocorrected your words to something nonsensical, you know firsthand that technology’s ability to recognize and understand language is imperfect. Some errors can be amusing, but others can have serious consequences. In 2017, automatic translation software translated a Palestinian man’s “good morning” post on Facebook as “attack them” in Hebrew and “hurt them” in English. The man was arrested and held by the police until the misunderstanding was resolved.
“That situation could have been even worse,” says Emily M. Bender, Howard and Frances Nostrand Endowed Professor in the Department of Linguistics, who is troubled by such errors. Bender is director of the UW’s Computational Linguistics program and specializes in natural language processing (NLP), which she defines as “everything involved with getting computers to handle human languages.” For the past few years, she has explored ethical issues related to NLP.
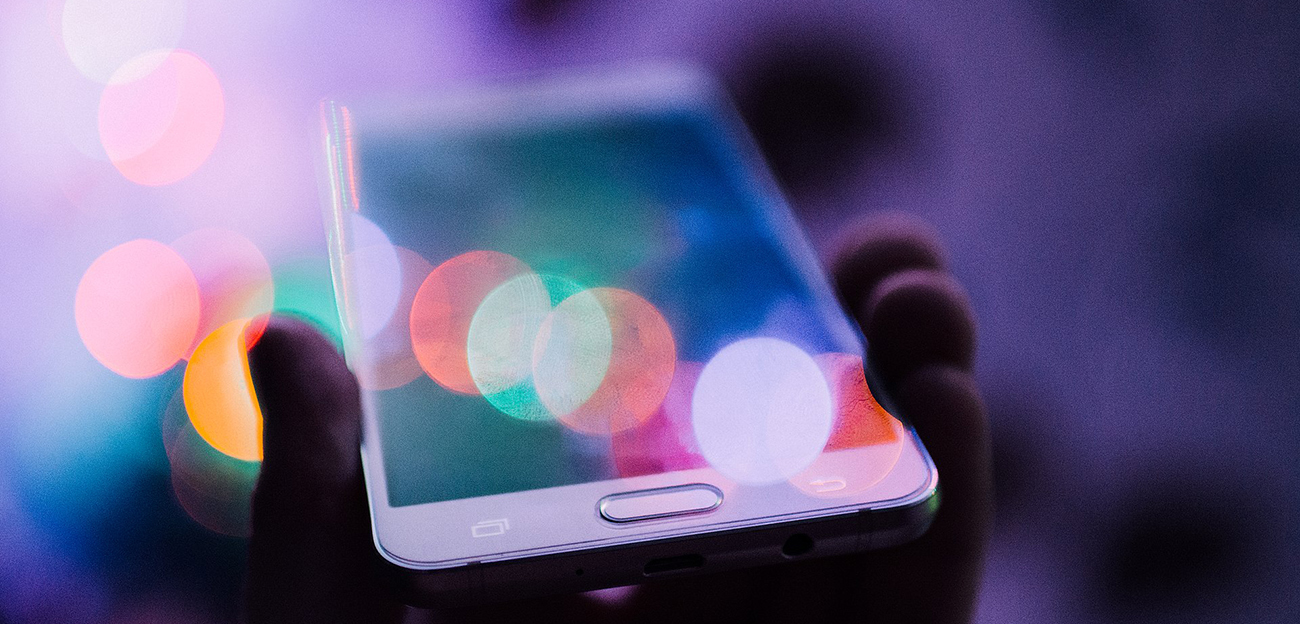
NLP systems do not magically understand human language. They must be trained. Developers use training data — for speech recognition, that would be things like transcribed recordings of speakers or captioned videos — in hopes of building systems that will accurately process language from a wide range of speakers. But those training data cannot possibly reflect all possible variations in speech, including every accent, dialect, and slang expression. When NLP technology is confronted with an unfamiliar word or sound, it will guess at the correct meaning, which can lead to errors.
As language technology has evolved, we are seeing new kinds of problems. Most early speech recognition involved a very small number of possible words — yes, no, a few numbers — that worked across many speakers (who might be asked to “press or say 1” at the start of a phone call, for example). At the same time, other NLP programs enabled individual users to train the system to understand their specific speech patterns, so they could replace a keyboard with speech recognition. While the first approach involved many speakers but few words, the second involved a single speaker but many words. Today we expect NLP to combine those two approaches, deftly processing a wide range of words but also representing a broad range of users, which is why the quality of training data has become so important.
NLP is not magic, it’s just a guess based on patterns in some set of training data.
“Companies developing language technologies use whatever they can get their hands on for training data,” says Bender. “Some of it is likely to be commissioned. In the speech recognition example, this would be people paid to read existing text, but a bunch of it might also be from captioned videos or other user-generated content. It’s not going to be broadly representative of everybody’s speech varieties, especially if it isn’t collected systematically. When the speech recognition system doesn’t have data that matches its users, there are going to be problems. How bad the problems are depends on what the system is being used for. As far as I know, no one has put speech recognition in a 911 system yet, but if they did, it could be disastrous.”
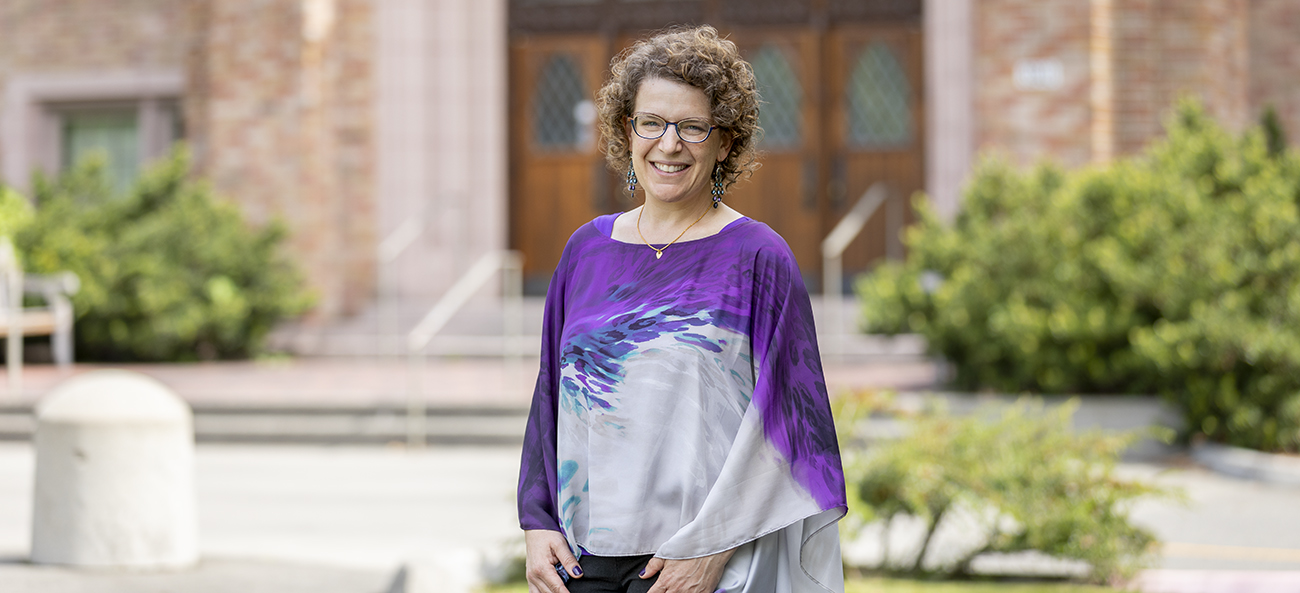
Bender views this as an ethical issue, since groups underrepresented in training data are at risk of being misrepresented or worse. She has identified other NLP ethics issues as well, one of the most obvious being the bias inherent in gendered virtual assistants like Siri and Alexa, which are programmed to remain cheerful while we bark orders at them. There are also issues with NLP’s ability to profile users based on their language characteristics — information that might be shared with advertisers to target specific audiences or used for surveillance. The list of concerns goes on and on.
Several years ago, eager to integrate ethics into the NLP curriculum, Bender searched for an expert to teach a graduate seminar on ethics and natural language processing. The topic was so new that there was no expert, so she researched and taught the course herself. She is offering it again this quarter.
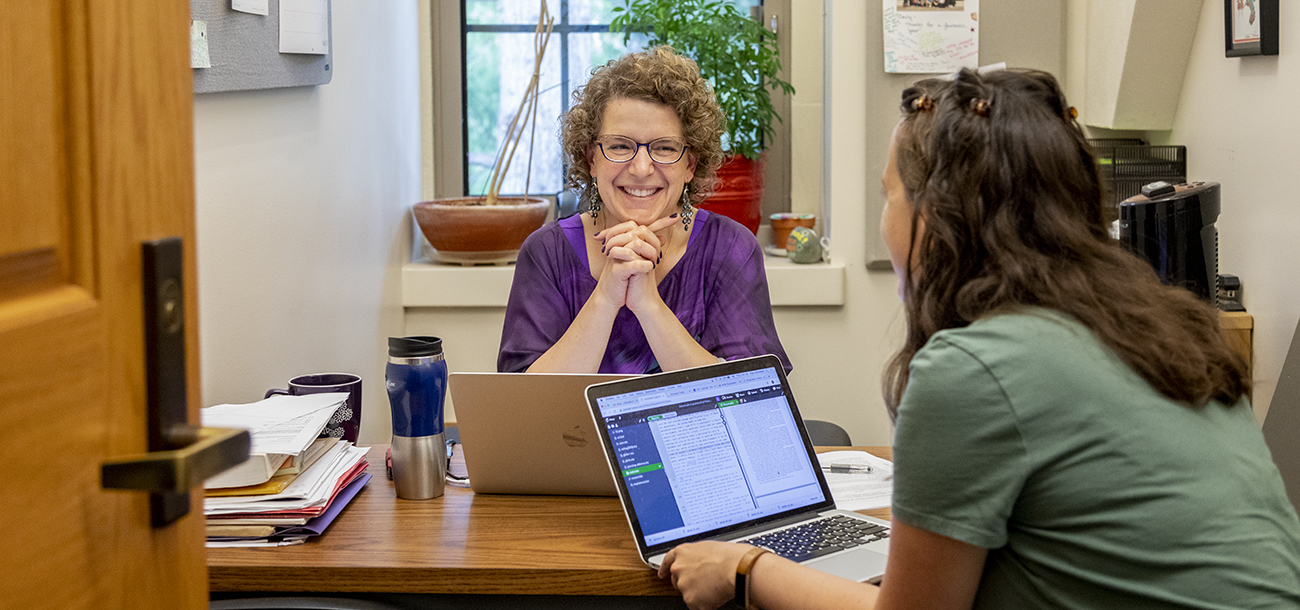
One topic covered is value sensitive design, a set of techniques for connecting the technology we build with the values of the people it will impact — essentially addressing the human consequences of a new technology. Bender invited UW iSchool professor Batya Friedman, a key figure in value sensitive design, to speak to her class. She found the talk inspiring. “Most of the readings I had put together for that class were, ‘Here are the problems. Here’s what’s bad. Here’s what we have to worry about,’” says Bender. “Value sensitive design is proactive. It’s ‘here’s what we can do about it.’”
Friedman and Bender are now collaborating to expand on that idea. They have developed “data statements,” through which NLP developers can provide detailed demographic information about the training data used for their NLP system, along with the rationale for that choice of data. (The data set might skew toward young adults, for example, if that is the intended user audience.) The hope is that by preparing a data statement, developers will give greater thought to direct users and indirect stakeholders — others affected by the technology — and provide greater transparency throughout the process.
...we need developers trained in the humanistic fields, who understand how people move and interact in the world.
That transparency extends to end users, many of whom are too trusting of NLP-generated information. “NLP is not magic, it’s just a guess based on patterns in some set of training data,” says Bender. “The more that we can make people aware that it’s just a guess and not necessarily accurate, I think the better positioned and informed they will be about how to make use of this kind of technology.”
Since Bender’s first seminar on ethics and NLP, ethics topics have been added to courses across the computational linguistics curriculum in the Department of Linguistics. Bender believes that linguists and others in the humanities and humanistic social sciences can — and must — play an important role in this ongoing work.
“If we are going to build computer technology that serves people, that supports people, that is pro-social, it needs to be built with an understanding of not just users but of other indirect stakeholders who get impacted,” she says. “For that we need developers trained in the humanistic fields, who understand how people move and interact in the world. That must inform the design of computer systems that are going to have a large impact on the world, or those systems are not going to help people.”
More Stories
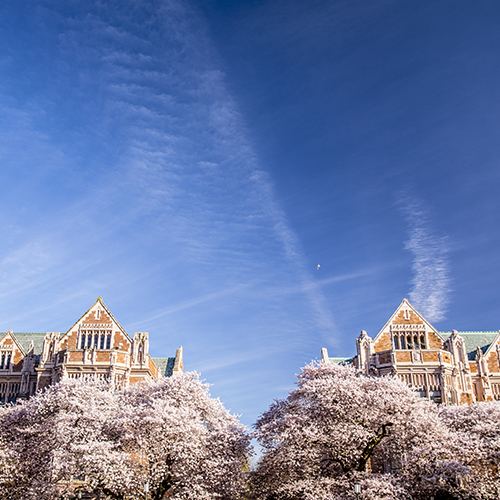
Meet Our 2025 Graduate Medalists
Meet the four graduating students selected by the College of Arts & Sciences as 2025 Graduate Medalists for their accomplishments.
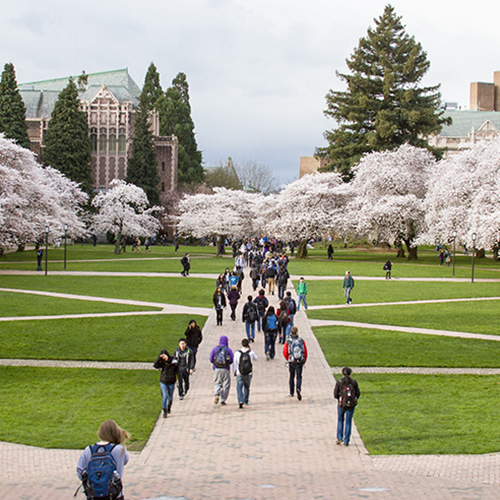
2025 Dean's Medalists, Energized & Inspiring
Meet the four new graduates honored as College of Arts & Sciences Dean's Medalists for 2025.
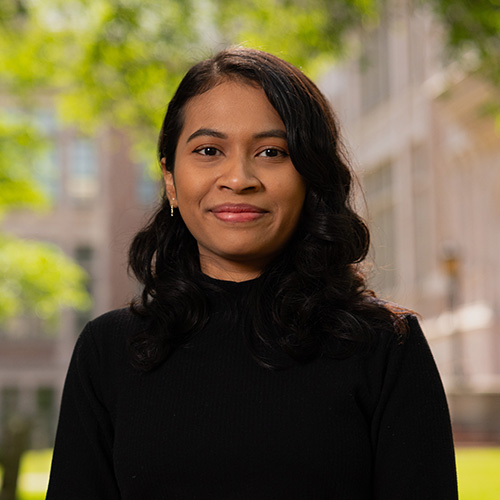
Balancing Sci-Fi and Scholarship
Speculative fiction author Anselma Prihandita (PhD, language and rhetoric, 2025), a Nebula Award winner, finds that her creative writing bolsters her scholarly work in unexpected ways.