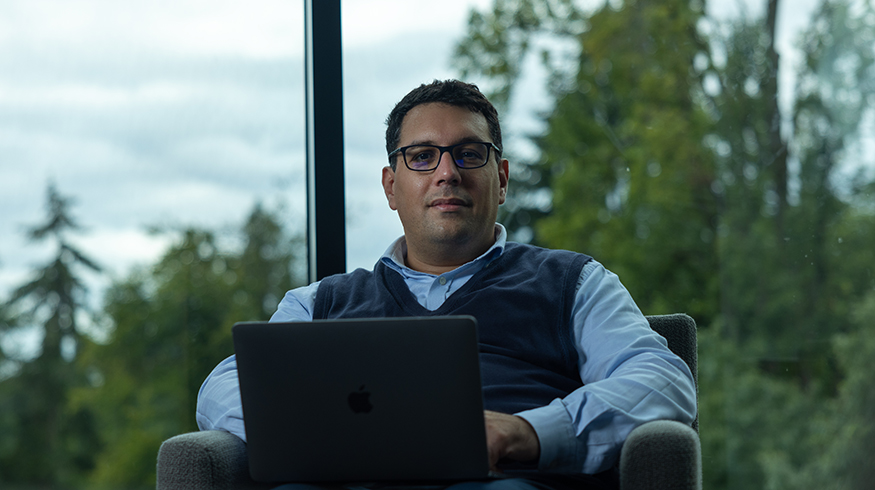
When ChatGPT launched, the world marveled at what it could do. Artificial intelligence (AI) has been around for decades, but suddenly it felt more accessible. Anyone could use ChatGPT — or similar virtual assistants that followed — to create artwork or write a professional cover letter or develop a training program for their child’s soccer team.
Zaid Harchaoui, professor of statistics in the College of Arts & Sciences and adjunct professor in the Paul G. Allen School of Computer Science & Engineering in the College of Engineering, has been more circumspect than most about these recent AI models. Harchaoui focuses on research at the intersection of statistics and computing, with an emphasis on machine learning. Collaborating with colleagues in statistics, mathematics, computer science, and engineering, he explores what AI models do well, where they fall short, and why.
You’ve been studying ChatGPT and similar AI models. What led to that focus?
When ChatGPT came out, the vast majority of people had never interacted with such a thing. When they submitted questions to it, the answers were so mind-blowing that some people started to attribute human-like features to it. But such incidental interactions can be misleading. This is far from a comprehensive and systematic evaluation. Jumping to the conclusion so quickly after a handful of interactions is a superstitious and dangerous thing to do. Even some colleagues were dazzled by the performance of ChatGPT. Many research scientists and engineers lost the usual skepticism that is natural to any scientist in the face of scientific and technological progress. It’s a gigantic endeavor to rigorously test the system, but I thought it was important to do that and see how it actually performs.
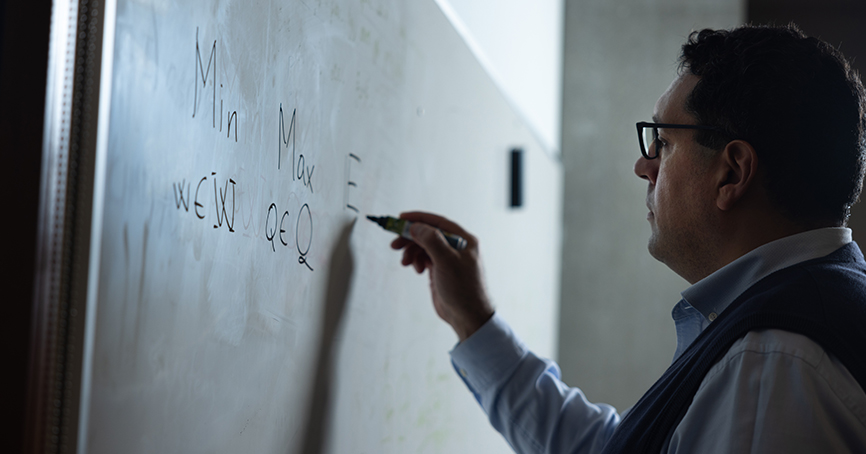
What did you find?
We’re finding that the range of expertise of AI models is different from our range of expertise as humans when it comes to problem solving. AI models can solve some things quickly, but there are many things that human beings consider easy that those machines will fail miserably at. An example is multiplying numbers. Kids, once they understand the rules, can do multiplication well. But AI models seem to struggle with that. Or if several people are crossing the street and one is dressed in gray, a self-driving car with AI might identify that person as a utility pole. That's the kind of mistake that a machine may make that is rare but would be extremely dangerous.
What is causing machines to struggle with some simple tasks?
We don’t know yet. It’s an active research topic, how to make machines more capable to solve a larger set of problems. A decade ago, the gap between mathematical understanding of machine learning and practical applications was not that wide. But as processors for computers have become more powerful and the data we can collect from the internet has exploded, people have been able to create much more complex models that can learn from data without having a mathematical understanding of the models. As a result, many recent advances like ChatGPT or CLIP were developed by trial and error, with little mathematical understanding of how they are actually tackling the problems.
Trial and error sounds wildly inefficient for AI projects on this scale. How did these projects progress so quickly?
ChatGPT uses a popular AI model called the transformer model. In the early 1990s, one component1 of this model — an attention module that mimics how human attention works, assigning varying levels of importance to input data — was shown to perform well.2 Around 2017, a group of researchers built a model that just iterates that component, basically like a chain of that same component, and found that when they did that, they got a model that performs really well.3 Nobody knows why. I'm currently working on better understanding this statistically.
...it's incredible that such large amounts of resources are dedicated to an extremely ambitious pursuit with such a lack of diversity in approaches. It's just a strange dynamic.
What about other AI models?
In AI right now, everybody is using the same approach — the transformer model — at a very large scale. People are betting that with more computing and more data and larger models, at some point a big intelligence will arise. Maybe it's true. I don't know. But most likely, the lack of alternative approaches will become a big problem. I don't know what will happen, but it's incredible that such large amounts of resources are dedicated to an extremely ambitious pursuit with such a lack of diversity in approaches. It's just a strange dynamic.
After ChatGPT was released, some of its shortcomings emerged. Do you think it was released before it was ready?

It was a spectacular experiment on a planetary scale. Everyone could test and experiment with it. This reflected a radically new proposal for the transparent development of AI. The whole industry had to quickly align with that peculiar approach. Many of the big industry players had to catch up and release things earlier than they would have preferred. As a result, most of the large models that are currently released are far from being ready as products. They can be very clumsy.
In terms of your own work with AI, what is your end goal?
The current process to produce AI systems is expensive. People say that large corporations by 2025 will be able to design models that have used all the data from the internet, which seems like expensive data usage just to understand simple things that we ask those models to do. The number of GPUs [graphical processing units] and the cloud computing we use for this is just crazy. It's wonderful that we've made all this progress, demonstrating that it was possible with such a debauchery of means — large computing, large data — but now I think we should also try to understand how those models work, what data was really useful and so on, so that we can achieve the same thing with much more economical means. But for me, it's not really about the means. It's more about elegance. In mathematics and statistics, there are proofs that are pedestrian and tedious with many steps and proofs that consist of a few steps and are elegant. So there is some aesthetic challenge that can be shaped by analyzing the current models and understanding why they work.
You’re part of an interdisciplinary team that’s doing this work. How important is it to have an interdisciplinary approach?
AI endeavors involve efforts that are conceptual — coming up with concepts to explain what's going on, which is more on the mathematical/statistical side — as well as efforts that are more on the engineering side, which is why I collaborate with people in applied AI domains. But it doesn't necessarily mean that collaborative research is compartmentalized that way. There are times when I can be the one contributing more to the applied AI side and they are contributing more to the theoretical foundations side. It’s wonderful when it happens, because you really feel like it is an intellectual endeavor and the boundaries are irrelevant. The UW is the perfect environment for this because the University has a culture of collaboration. When people propose collaborative projects at the UW, everybody's excited.
What are your thoughts on the future of AI?
I think at some point AI will reach a high peak, meaning it will be good at many more things. But it will still not be good at other things. And then, even with more data and more computers, it won’t do much better. Hopefully then people will get back to the workbench and come up with new ideas. I think it's important to maintain a plurality and a diversity of approaches, and to try to understand what's going on so we can come up with those new ideas and simplify what we've been doing so far.
1 The component that is repeatedly used in transformer models is an attention module. Attention modules mimic how human attention works by assigning varying levels of importance to different parts or sections of input data.
2 Schlag and Schmidhuber. Linear Transformers Are Secretly Fast Weight Programmers, PMLR, 2021.
3 Vaswani et al. Attention is all you need, Neurips, 2017.
For further reading, here are articles from Zaid Harchaoui's recent collaborative multi-disciplinary projects:
Pillutla, Liu, et al. Mauve Scores for Generative Models: Theory and Practice. JMLR 2023.
Dziri, Lu, Sclar, et al. Faith and Fate: Limits of Transformers on Compositionality, Neurips 2023.
Liu, Mehta, et al. The Benefits of Balance: From Information Projections to Variance Reduction. arXiv 2024.
More Stories
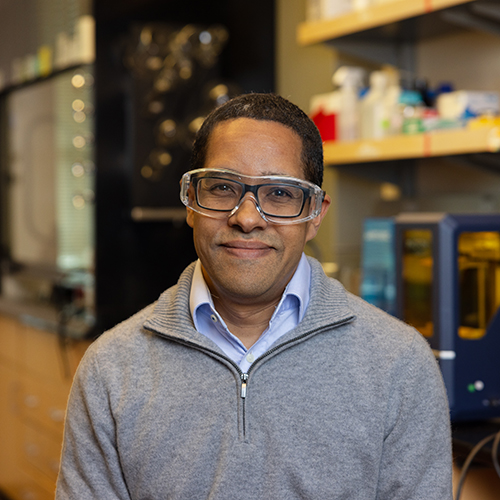
Through Chemistry and 3D Printing, New Materials Emerge
Chemistry professor Alshakim Nelson and his research team use 3D-printing technology to develop new materials with potential real-world applications in medicine, engineering, and sustainability.
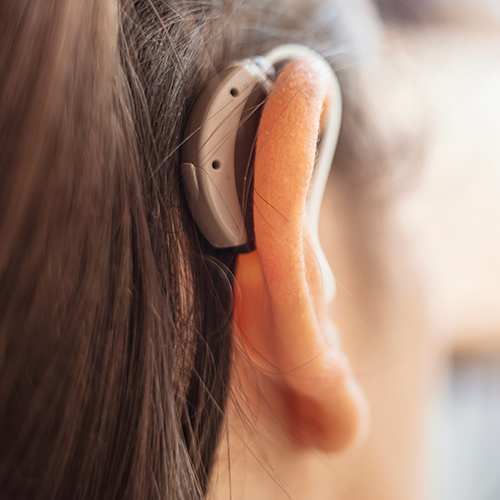
An Earful of AI
Hearing aid technology is improving all the time with the help of AI, thanks to researchers like Yi Shen, professor of speech & hearing sciences.
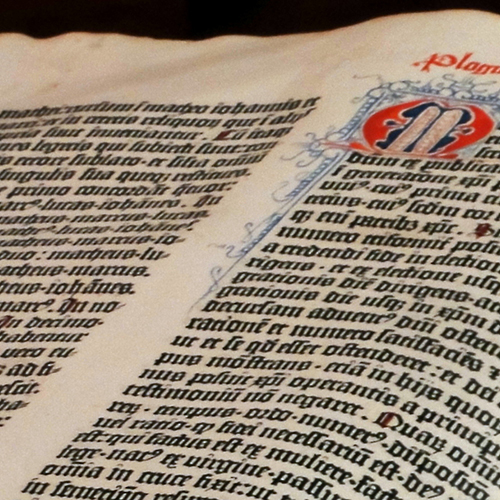
The Evolution of the Book
As books evolve with new technologies, Geoffrey Turnovsky, professor of French, explores the history of texts — and the reading experience.